He has research experience in the area of health services research with a focus on chronic illnesses, cancer and mental health in particular. His current research interests are in the area of Post-Traumatic Stress Disorder while continuing to publish in the cancer field. As a psychologist he treats adults in a private clinic and also students at the University of Newcastle.
He is a Member of the Australian Psychological Society and is currently writing his second book with Dr Law on managing mood. His wife Asiyah and daughter Gabriela live in Newcastle Australia. Lorem ipsum dolor sit amet, consectetur adipisicing elit, sed do eiusmod tempor incididunt ut labore et dolore magna aliqua. Close Find out more. Main Description Statistics are a vital skill for epidemiologists and form an essential part of clinical medicine and public health. Mathematical and Statistical Symbols II: Foundations of Epidemiology 2: For example, it appears to be less important for cancer in general than for chronic obstructive lung disease.
The healthy worker effect in a given group of workers tends to diminish over time. Sometimes a complete tabulation of a cohort i. Computation of person-years requires special methods to deal with person-time assessment, including life-table methods. Without total person-time information on all cohort members, regardless of disease status, SMRs and SRRs cannot be calculated. Instead, proportional mortality ratios PMRs can be used. A PMR is the ratio of the observed number of deaths due to a specific cause in comparison to the expected number, based on the proportion of total deaths due to the specific cause in the referent population, multiplied by the number of total deaths in the study group, multiplied by Similarly, some apparent deficits may merely reflect real excesses of other causes of death.
For example, if aerial pesticide applicators have a large real excess of deaths due to accidents, the mathematical requirement that the PMR for all causes combined equal may cause some one or other causes of death to appear deficient even if the mortality is excessive. To ameliorate this potential problem, researchers interested primarily in cancer can calculate proportionate cancer mortality ratios PCMRs. PCMRs compare the observed number of cancer deaths to the number expected based on the proportion of total cancer deaths rather than all deaths for the cancer of interest in the referent population multiplied by the total number of cancer deaths in the study group, multiplied by Thus, the PCMR will not be affected by an aberration excess or deficit in a non-cancer cause of death, such as accidents, heart disease or non-malignant lung disease.
PMR studies can better be analysed using mortality odds ratios MORs , in essence analysing the data as if they were from a case-control study. For example, if the main interest of the study were cancer, mortality odds ratios could be calculated comparing exposure among the cancer deaths to exposure among the cardiovascular deaths. This approach, like the PCMR, avoids the problems with the PMR which arise when a fluctuation in one cause of death affects the apparent risk of another simply because the overall PMR must equal The choice of the control causes of death is critical, however.
As mentioned above, they must not be related to the exposure, but the possible relationship between exposure and disease may not be known for many potential control diseases. There are measures available which express the amount of disease that would be attributable to an exposure if the observed association between the exposure and disease were causal. The attributable risk in the exposed AR e is the disease rate in the exposed minus the rate in the unexposed. Because disease rates cannot be measured directly in case-control studies, the AR e is calculable only for cohort studies.
The PAR can be obtained from cohort studies table There are several measures of risk that have been described. Each assumes underlying methods for counting events and in the representatives of these events to a defined group. When results are compared across studies, an understanding of the methods used is essential for explaining any observed differences. The epidemiologist is interested in relationships between variables, chiefly exposure and outcome variables. Typically, epidemiologists want to ascertain whether the occurrence of disease is related to the presence of a particular agent exposure in the population.
The ways in which these relationships are studied may vary considerably. One can identify all persons who are exposed to that agent and follow them up to measure the incidence of disease, comparing such incidence with disease occurrence in a suitable unexposed population. Alternatively, one can simply sample from among the exposed and unexposed, without having a complete enumeration of them. Follow-up of study participants is one option in so-called longitudinal studies: One alternative option is a cross-section of the population, where both exposure and disease are measured at the same point in time.
Search form
To set the stage for this discussion, consider a large viscose rayon factory in a small town. An investigation into whether carbon disulphide exposure increases the risk of cardiovascular disease is started. The investigation has several design choices, some more and some less obvious. A first strategy is to identify all workers who have been exposed to carbon disulphide and follow them up for cardiovascular mortality. A cohort study encompasses research participants sharing a common event, the exposure. At entrance, all cohort members should be free of the disease under study, according to the empirical set of criteria used to measure the disease.
If, for example, in the cohort study on the effects of carbon disulphide on coronary morbidity, coronary heart disease is empirically measured as clinical infarctions, those who, at the baseline, have had a history of coronary infarction must be excluded from the cohort. By contrast, electrocardiographic abnormalities without a history of infarction can be accepted. However, if the appearance of new electrocardiographic changes is the empirical outcome measure, the cohort members should also have normal electrocardiograms at the baseline.
The morbidity in terms of incidence or the mortality of an exposed cohort should be compared to a reference cohort which ideally should be as similar as possible to the exposed cohort in all relevant aspects, except for the exposure, to determine the relative risk of illness or death from exposure. Using a similar but unexposed cohort as a provider of the reference experience is preferable to the common mal practice of comparing the morbidity or mortality of the exposed cohort to age-standardized national figures, because the general population falls short on fulfilling even the most elementary requirements for comparison validity.
The Standardized Morbidity or Mortality Ratio SMR , resulting from such a comparison, usually generates an underestimate of the true risk ratio because of a bias operating in the exposed cohort, leading to the lack of comparability between the two populations. On the other hand, the cohort design permits the study of several diseases at the same time.
Epidemiology
While well-suited to test specific hypotheses e. Although the study was to determine if foundry dust causes lung cancer, the data source, with the same effort, also gives information on all other causes of death. Therefore, other possible health risks can be studied at the same time. The timing of a cohort study can either be retrospective historical or prospective concurrent. In both instances the design structure is the same.
A full enumeration of exposed people occurs at some point or period in time, and the outcome is measured for all individuals through a defined end point in time. The difference between prospective and retrospective is in the timing of the study. If retrospective, the end point has already occurred; if prospective, one has to wait for it.
In the retrospective design, the cohort is defined at some point in the past for example, those exposed on 1 January , or those taking on exposed work between and However, the more complete the follow-up, the more valid is the study. In the prospective design, the cohort is defined at the present, or during some future period, and the morbidity is then followed into the future.
When doing cohort studies, enough time must be allowed for follow-up in order that the end points of concern have sufficient time to manifest. Sometimes, because historical records may be available for only a short period into the past, it is nevertheless desirable to take advantage of this data source because it means that a shorter period of prospective follow-up would be needed before results from the study could be available. In these situations, a combination of the retrospective and the prospective cohort study designs can be efficient.
The general layout of frequency tables presenting cohort data is shown in table N 0 and N 1 are usually expressed in person-time units instead of as the number of people in the populations.
- Punished With Sex: Hot for Hitchhikers Volume One?
- Where we work?
- Figuring Out People;
Person-years are computed for each individual separately. Different people often enter the cohort during a period of time, not at the same date. Hence their follow-up times start at different dates. If the RR is greater than 1, the morbidity of the exposed cohort is higher than that of the reference cohort, and vice versa.
The RR is a point estimate and a confidence interval CI should be computed for it. The larger the study, the narrower the confidence interval will become. Also for the SMR, a confidence interval should be computed. It is better to give this measure in a publication than a p-value, because statistical significance testing is meaningless if the general population is the reference category. Such comparison entails a considerable bias the healthy worker effect noted above , and statistical significance testing, originally developed for experimental research, is misleading in the presence of systematic error.
Suppose the question is whether quartz dust causes lung cancer. Granite quarries do not expose the stone workers to these other carcinogens. Therefore the problem is best studied among stone workers employed in granite quarries. Suppose then that all 2, workers, having been employed by 20 quarries between and , are enrolled in the cohort and their cancer incidence alternatively only mortality is followed starting at ten years after first exposure to allow for an induction time and ending in This is a to year depending on the year of entry or, say, on average, a year follow-up of the cancer mortality or morbidity among 1, of the quarry workers who were specifically granite workers.
The exposure history of each cohort member must be recorded. Those who have left the quarries must be traced and their later exposure history recorded. In countries where all inhabitants have unique registration numbers, this is a straightforward procedure, governed chiefly by national data protection laws. Where no such system exists, tracing employees for follow up purposes can be extremely difficult. Where appropriate death or disease registries exist, the mortality from all causes, all cancers and specific cancer sites can be obtained from the national register of causes of death.
For cancer mortality, the national cancer registry is a better source because it contains more accurate diagnoses. In addition, incidence or, morbidity data can also be obtained. Suppose that 70 fatal cases of lung cancer are found in the cohort, whereas the expected number the number which would have occurred had there been no exposure is If detailed exposure data are available, the cancer mortality can be studied as a function of different latency times say, 10, 15, 20 years , work in different types of quarries different kinds of granite , different historical periods, different exposure intensities and so on.
However, 70 cases cannot be subdivided into too many categories, because the number falling into each one rapidly becomes too small for statistical analysis. Both types of cohort designs have advantages and disadvantages. A retrospective study can, as a rule, measure only mortality, because data for milder manifestations usually are lacking.
Cancer registries are an exception, and perhaps a few others, such as stroke registries and hospital discharge registries, in that incidence data also are available. Assessing past exposure is always a problem and the exposure data are usually rather weak in retrospective studies. This can lead to effect masking. On the other hand, since the cases have already occurred, the results of the study become available much sooner; in, say, two to three years.
Several different manifestations of a disease can be measured. Measurements of both exposure and outcome can be repeated, and all measurements can be standardized and their validity can be checked. Much can happen during this time. For example, turnover of researchers, improvements in techniques for measuring exposure, remodelling or closure of the plants chosen for study and so forth.
All these circumstances endanger the success of the study. The costs of a prospective study are also usually higher than those of a retrospective study, but this is mostly due to the much greater number of measurements repeated exposure monitoring, clinical examinations and so on , and not to more expensive death registration. Therefore the costs per unit of information do not necessarily exceed those of a retrospective study.
In view of all this, prospective studies are more suited for diseases with rather short latency, requiring short follow-up, while retrospective studies are better for disease with a long latency. Let us go back to the viscose rayon plant. A retrospective cohort study may not be feasible if the rosters of the exposed workers have been lost, while a prospective cohort study would yield sound results in a very long time.
An alternative would then be the comparison between those who died from coronary heart disease in the town, in the course of a defined time period, and a sample of the total population in the same age group. The classical case-control or, case-referent design is based on sampling from a dynamic open, characterized by a turnover of membership population. This population can be that of a whole country, a district or a municipality as in our example , or it can be the administratively defined population from which patients are admitted to a hospital.
The defined population provides both the cases and the controls or referents. The technique is to gather all the cases of the disease in question that exist at a point in time prevalent cases , or have occurred during a defined period of time incident cases. The cases thus can be drawn from morbidity or mortality registries, or be gathered directly from hospitals or other sources having valid diagnostics. The controls are drawn as a sample from the same population, either from among non-cases or from the entire population.
Another option is to select patients with another disease as controls, but then these patients must be representative of the population from which the cases came. There may be one or more controls i. The sampling approach differs from cohort studies, which examine the entire population. It goes without saying that the gains in terms of the lower costs of case-control designs are considerable, but it is important that the sample is representative of the whole population from which the cases originated i.
When cases and controls have been identified, their exposure histories are gathered by questionnaires, interviews or, in some instances, from existing records e. The data can be obtained either from the participants themselves or, if they are deceased, from close relatives. To ensure symmetrical recall, it is important that the proportion of dead and live cases and referents be equal, because close relatives usually give a less detailed exposure history than the participants themselves.
Information about the exposure pattern among cases is compared to that among controls, providing an estimate of the odds ratio OR , an indirect measure of the risk among the exposed to incur the disease relative to that of the unexposed. Because the case-control design relies on the exposure information obtained from patients with a certain disease i. By contrast, this design allows the concomitant study of the effect of several different exposures. The case-referent study is well suited to address specific research questions e. Cases of primary liver cancer, a comparatively rare disease in Europe, are best gathered from a national cancer registry.
- Theodore Boone: The Activist: Theodore Boone 4.
- Hooponopono. Conéctate con los milagros (Psicología y Autoayuda) (Spanish Edition)!
- Les sabates descordades (Catalan Edition).
- What I Remember: Eighty Years.
- Navigation menu.
- Statistical Epidemiology.
Suppose that all cancer cases occurring during three years form the case series. The population base for the study is then a three-year follow-up of the entire population in the European country in question. The controls are drawn as a sample of persons without liver cancer from the same population. For reasons of convenience meaning that the same source can be used for sampling the controls patients with another cancer type, not related to solvent exposure, can be used as controls.
Colon cancer has no known relation to solvent exposure; hence this cancer type can be included among the controls. Using cancer controls minimizes recall bias in that the accuracy of the history given by cases and controls is, on average, symmetrical. For each case of liver cancer, two controls are drawn in order to achieve greater statistical power.
One could draw even more controls, but available funds may be a limiting factor. If funds were not limited, perhaps as many as four controls would be optimal. Beyond four, the law of diminishing returns applies. After obtaining appropriate permission from data protection authorities, the cases and controls, or their close relatives, are approached, usually by means of a mailed questionnaire, asking for a detailed occupational history with special emphasis on a chronological list of the names of all employers, the departments of work, the job tasks in different employment, and the period of employment in each respective task.
These data can be obtained from relatives with some difficulty; however, specific chemicals or trade names usually are not well recalled by relatives. The questionnaire also should include questions on possible confounding data, such as alcohol use, exposure to foodstuffs containing aflatoxins, and hepatitis B and C infection. In order to obtain a sufficiently high response rate, two reminders are sent to non-respondents at three-week intervals. The ten years of exposure immediately preceding the cancer diagnosis are disregarded, because it is not biologically plausible that initiator-type carcinogens can be the cause of the cancer if the latency time is that short although promoters, in fact, could.
At this stage it is also possible to differentiate between different types of solvent exposure. Because a complete employment history has been given, it is also possible to explore other exposures, although the initial study hypothesis did not include these.
Odds ratios can then be computed for exposure to any solvent, specific solvents, solvent mixtures, different categories of exposure intensity, and for different time windows in relation to cancer diagnosis. It is advisable to exclude from analysis those with unknown exposure. The cases and controls can be sampled and analysed either as independent series or matched groups. Matching means that controls are selected for each case based on certain characteristics or attributes, to form pairs or sets, if more than one control is chosen for each case.
Matching is usually done based on one or more such factors, as age, vital status, smoking history, calendar time of case diagnosis, and the like. In our example, cases and controls are then matched on age and vital status. Vital status is important, because patients themselves usually give a more accurate exposure history than close relatives, and symmetry is essential for validity reasons.
Today, the recommendation is to be restrictive with matching, because this procedure can introduce negative effect-masking confounding. If one control is matched to one case, the design is called a matched-pair design. Provided the costs of studying more controls are not prohibitive, more than one referent per case improves the stability of the estimate of the OR, which makes the study more size efficient. The layout of the results of an unmatched case-control study is shown in table The estimate of the OR is then:. If relatively more cases than controls have been exposed, the OR is in excess of 1 and vice versa.
Confidence intervals must be calculated and provided for the OR, in the same manner as for the RR. By way of a further example, an occupational health centre of a large company serves 8, employees exposed to a variety of dusts and other chemical agents. We are interested in the connection between mixed dust exposure and chronic bronchitis.
The study involves follow-up of this population for one year. Each patient visiting the health centre and fulfilling these criteria during a one-year period is a case, and the next patient seeking medical advice for non-pulmonary problems is defined as a control. Suppose that cases and controls become enrolled during the study period. Let 40 cases and 15 controls be classified as having been exposed to dust. In the foregoing example, no consideration has been given to the possibility of confounding, which may lead to a distortion of the OR due to systematic differences between cases and controls in a variable like age.
One way to reduce this bias is to match controls to cases on age or other suspect factors. This results in a data layout depicted in table When both members of a pair are exposed or unexposed, the pair is disregarded. The OR in a matched-pair study design is defined as. In a study on the association between nasal cancer and wood dust exposure, there were all together case-control pairs.
In only one pair, both the case and the control had been exposed, and in pairs, neither the case nor the control had been exposed. These pairs are not further considered. The case, but not the control had been exposed in 12 pairs, and the control, but not the case, in one pair. Case-control studies are more efficient than cohort studies when the disease is rare; they may in fact provide the only option. However, common diseases also can be studied by this method. If the exposure is rare, an exposure-based cohort is the preferable or only feasible epidemiological design.
Of course, cohort studies also can be carried out on common exposures. The choice between cohort and case-control designs when both the exposure and disease are common is usually decided taking validity considerations into account. Selective recall can cause an effect-magnifying bias through differential asymmetrical misclassification of exposure status. The advantages of case-control studies lie in their cost-effectiveness and their ability to provide a solution to a problem relatively quickly. Because of the sampling strategy, they allow the investigation of very large target populations e.
In countries where data protection legislation or lack of good population and morbidity registries hinders the execution of cohort studies, hospital-based case-control studies may be the only practical way to conduct epidemiological research. A cohort study also can be designed for sampling instead of complete follow-up. A sampling approach within the cohort sets different requirements on cohort eligibility, because the comparisons are now made within the same cohort.
This should therefore include not only heavily exposed workers, but also less-exposed and even unexposed workers, in order to provide exposure contrasts within itself. It is important to realize this difference in eligibility requirements when assembling the cohort. However, provided the cohort has a broad range of exposure experience, the nested case-control approach is very attractive.
One gathers all the cases arising in the cohort over the follow-up period to form the case series, while only a sample of the non-cases is drawn for the control series. The controls can either be matched to the cases or they can be treated as an independent series. The sampling approach can be less costly compared to exhaustive information procurement on each member of the cohort. In particular, because only a sample of controls is studied, more resources can be devoted to detailed and accurate exposure assessment for each case and control.
However, the same statistical power problems prevail as in classical cohort studies. In a scientific sense, a cross-sectional design is a cross-section of the study population, without any consideration given to time. Both exposure and morbidity prevalence are measured at the same point in time. From the aetiological point of view, this study design is weak, partly because it deals with prevalence as opposed to incidence. Prevalence is a composite measure, depending both on the incidence and duration of the disease.
This also restricts the use of cross-sectional studies to diseases of long duration. Even more serious is the strong negative bias caused by the health-dependent elimination from the exposed group of those people more sensitive to the effects of exposure. Therefore aetiological problems are best solved by longitudinal designs. Indeed, cross-sectional studies do not permit any conclusions about whether exposure preceded disease, or vice versa.
The cross-section is aetiologically meaningful only if a true time relation exists between the exposure and the outcome, meaning that present exposure must have immediate effects. However, the exposure can be cross-sectionally measured so that it represents a longer past time period e. The study then is a mixture of a longitudinal and a cross-sectional design rather than a mere cross-section of the study population.
Cross-sectional surveys are often useful for practical and administrative, rather than for scientific, purposes. Epidemiological principles can be applied to systematic surveillance activities in the occupational health setting, such as:. It is important to choose representative, valid, and specific morbidity indicators for all types of surveys.
A survey or a screening programme can use only a rather small number of tests, in contrast to clinical diagnostics, and therefore the predictive value of the screening test is important.
University of London
Insensitive methods fail to detect the disease of interest, while highly sensitive methods produce too many falsely positive results. It is not worthwhile to screen for rare diseases in an occupational setting. All case finding i. Otherwise only frustration will result with a potential for more harm than good emerging.
Epidemiology aims at providing an understanding of the disease experience in populations. In particular, it can be used to obtain insight into the occupational causes of ill health. This knowledge comes from studies conducted on groups of people having a disease by comparing them to people without that disease.
- Captain Condom Book 03!
- Les Héros, ça strompe jamais, épisode 2 (Numerik séries) (French Edition).
- Credit Cheat Code 101: 4 Simple Tricks To Unlock Good Credit In Less Than 30 Days. Learn Forbidden Information That Banks, Creditors And FICO Doesnt Want ... (Forbidden Fruit Books: Credit Repair).
- Statistical epidemiology?
- Amazing Adventures, Volume 2, Monsters of Living Flame;
- Primary Menu;
Another approach is to examine what diseases people who work in certain jobs with particular exposures acquire and to compare these disease patterns to those of people not similarly exposed. These studies provide estimates of risk of disease for specific exposures. For information from such studies to be used for establishing prevention programmes, for the recognition of occupational diseases, and for those workers affected by exposures to be appropriately compensated, these studies must be valid.
Validity can be defined as the ability of a study to reflect the true state of affairs. A valid study is therefore one which measures correctly the association either positive, negative or absent between an exposure and a disease. It describes the direction and magnitude of a true risk. Two types of validity are distinguished: Validity relates to the truthfulness of a measurement. Validity must be distinguished from precision of the measurement, which is a function of the size of the study and the efficiency of the study design.
A study is said to be internally valid when it is free from biases and therefore truly reflects the association between exposure and disease which exists among the study participants. An observed risk of disease in association with an exposure may indeed result from a real association and therefore be valid, but it may also reflect the influence of biases. A bias will give a distorted image of reality. Three major types of biases, also called systematic errors, are usually distinguished:.
They will be presented briefly below, using examples from the occupational health setting. Selection bias will occur when the entry into the study is influenced by knowledge of the exposure status of the potential study participant. This problem is therefore encountered only when the disease has already taken place by the time before the person enters the study. Typically, in the epidemiological setting, this will happen in case-control studies or in retrospective cohort studies.
This means that a person will be more likely to be considered a case if it is known that he or she has been exposed. Three sets of circumstances may lead to such an event, which will also depend on the severity of the disease. This can occur when people who know they have been exposed to known or believed harmful products in the past and who are convinced their disease is the result of the exposure will consult a physician for symptoms which other people, not so exposed, might have ignored. This is particularly likely to happen for diseases which have few noticeable symptoms. An example may be early pregnancy loss or spontaneous abortion among female nurses handling drugs used for cancer treatment.
These women are more aware than most of reproductive physiology and, by being concerned about their ability to have children, may be more likely to recognize or label as a spontaneous abortion what other women would only consider as a delay in the onset of menstruation. Another example from a retrospective cohort study, cited by Rothman , involves a Centers for Disease Control study of leukaemia among troops who had been present during a US atomic test in Nevada.
This will occur when the doctors are more likely to diagnose a given disease once they know to what the patient has been previously exposed. Knowing the occupation of the patient made it easier to diagnose the disease even in its early stages, whereas the identification of the causal agent would be much more difficult in research participants not known to be occupationally exposed to lead.
When people, either healthy or sick, are asked to participate in a study, several factors play a role in determining whether or not they will agree. Willingness to answer variably lengthy questionnaires, which at times inquire about sensitive issues, and even more so to give blood or other biological samples, may be determined by the degree of self-interest held by the person.
Someone who is aware of past potential exposure may be ready to comply with this inquiry in the hope that it will help to find the cause of the disease, whereas someone who considers that they have not been exposed to anything dangerous, or who is not interested in knowing, may decline the invitation to participate in the study. This can lead to a selection of those people who will finally be the study participants as compared to all those who might have been.
This is also called observation bias and concerns disease outcome in follow-up studies and exposure assessment in case-control studies. Two groups are defined at the start of the study: Problems of diagnostic bias will arise if the search for cases differs between these two groups. For example, consider a cohort of people exposed to an accidental release of dioxin in a given industry.
For the highly exposed group, an active follow-up system is set up with medical examinations and biological monitoring at regular intervals, whereas the rest of the working population receives only routine care. It is highly likely that more disease will be identified in the group under close surveillance, which would lead to a potential over-estimation of risk.
The reverse mechanism to that described in the preceding paragraph may occur in retrospective cohort studies. In these studies, the usual way of proceeding is to start with the files of all the people who have been employed in a given industry in the past, and to assess disease or mortality subsequent to employment. Unfortunately, in almost all studies files are incomplete, and the fact that a person is missing may be related either to exposure status or to disease status or to both.
For example, in a recent study conducted in the chemical industry in workers exposed to aromatic amines, eight tumours were found in a group of workers who had undergone cytological screening for urinary tumours. Altogether, only 34 records were found missing, corresponding to a 4. This shows that the files of people who became cases were more likely to become lost than the files of other workers.
This may occur because of more frequent job changes within the company which may be linked to exposure effects , resignation, dismissal or mere chance. In case-control studies, the disease has already occurred at the start of the study, and information will be sought on exposures in the past. The subdiscipline of forensic epidemiology is directed at the investigation of specific causation of disease or injury in individuals or groups of individuals in instances in which causation is disputed or is unclear, for presentation in legal settings.
Small Navigation Menu
Epidemiological practice and the results of epidemiological analysis make a significant contribution to emerging population-based health management frameworks. Modern population-based health management is complex, requiring a multiple set of skills medical, political, technological, mathematical etc. This task requires the forward looking ability of modern risk management approaches that transform health risk factors, incidence, prevalence and mortality statistics derived from epidemiological analysis into management metrics that not only guide how a health system responds to current population health issues, but also how a health system can be managed to better respond to future potential population health issues.
Each of these organizations use a population-based health management framework called Life at Risk that combines epidemiological quantitative analysis with demographics, health agency operational research and economics to perform:. Applied epidemiology is the practice of using epidemiological methods to protect or improve the health of a population. Applied field epidemiology can include investigating communicable and non-communicable disease outbreaks, mortality and morbidity rates, and nutritional status, among other indicators of health, with the purpose of communicating the results to those who can implement appropriate policies or disease control measures.
As the surveillance and reporting of diseases and other health factors becomes increasingly difficult in humanitarian crisis situations, the methodologies used to report the data are compromised. One study found that less than half Among the mortality surveys, only 3. As nutritional status and mortality rates help indicate the severity of a crisis, the tracking and reporting of these health factors is crucial.
Vital registries are usually the most effective ways to collect data, but in humanitarian contexts these registries can be non-existent, unreliable, or inaccessible. As such, mortality is often inaccurately measured using either prospective demographic surveillance or retrospective mortality surveys.
Prospective demographic surveillance requires lots of manpower and is difficult to implement in a spread-out population. Retrospective morality surveys are prone to selection and reporting biases. Other methods are being developed, but are not common practice yet. Different fields in epidemiology have different levels of validity. One way to assess the validity of findings is the ratio of false-positives claimed effects that are not correct to false-negatives studies which fail to support a true effect.
To take the field of genetic epidemiology, candidate-gene studies produced over false-positive findings for each false-negative. By contrast genome-wide association appear close to the reverse, with only one false positive for every or more false-negatives. By contrast other epidemiological fields have not required such rigorous reporting and are much less reliable as a result. Random error is the result of fluctuations around a true value because of sampling variability. Random error is just that: It can occur during data collection, coding, transfer, or analysis.
Examples of random error include: Random error affects measurement in a transient, inconsistent manner and it is impossible to correct for random error. There is random error in all sampling procedures. This is called sampling error. Precision in epidemiological variables is a measure of random error. Precision is also inversely related to random error, so that to reduce random error is to increase precision. Confidence intervals are computed to demonstrate the precision of relative risk estimates.
The narrower the confidence interval, the more precise the relative risk estimate. There are two basic ways to reduce random error in an epidemiological study. The first is to increase the sample size of the study. In other words, add more subjects to your study. The second is to reduce the variability in measurement in the study. This might be accomplished by using a more precise measuring device or by increasing the number of measurements.
Note, that if sample size or number of measurements are increased, or a more precise measuring tool is purchased, the costs of the study are usually increased. There is usually an uneasy balance between the need for adequate precision and the practical issue of study cost. A systematic error or bias occurs when there is a difference between the true value in the population and the observed value in the study from any cause other than sampling variability.
An example of systematic error is if, unknown to you, the pulse oximeter you are using is set incorrectly and adds two points to the true value each time a measurement is taken. The measuring device could be precise but not accurate. Because the error happens in every instance, it is systematic. Conclusions you draw based on that data will still be incorrect.
Chapter 28 - Epidemiology and Statistics
But the error can be reproduced in the future e. A mistake in coding that affects all responses for that particular question is another example of a systematic error. The validity of a study is dependent on the degree of systematic error. Validity is usually separated into two components:. Selection bias occurs when study subjects are selected or become part of the study as a result of a third, unmeasured variable which is associated with both the exposure and outcome of interest. Sackett D cites the example of Seltzer et al.
Information bias is bias arising from systematic error in the assessment of a variable. A typical example is again provided by Sackett in his discussion of a study examining the effect of specific exposures on fetal health: Confounding has traditionally been defined as bias arising from the co-occurrence or mixing of effects of extraneous factors, referred to as confounders, with the main effect s of interest.
The counterfactual or unobserved risk R A0 corresponds to the risk which would have been observed if these same individuals had been unexposed i. The true effect of exposure therefore is: Since the counterfactual risk R A0 is unobservable we approximate it using a second population B and we actually measure the following relations: Example assumes binary outcome and exposure variables. Some epidemiologists prefer to think of confounding separately from common categorizations of bias since, unlike selection and information bias, confounding stems from real causal effects.
To date, few universities offer epidemiology as a course of study at the undergraduate level. One notable undergraduate program exists at Johns Hopkins University , where students who major in public health can take graduate level courses, including epidemiology, their senior year at the Bloomberg School of Public Health.
Although epidemiologic research is conducted by individuals from diverse disciplines, including clinically trained professionals such as physicians, formal training is available through Masters or Doctoral programs including Master of Public Health MPH , Master of Science of Epidemiology MSc.
Many other graduate programs, e.
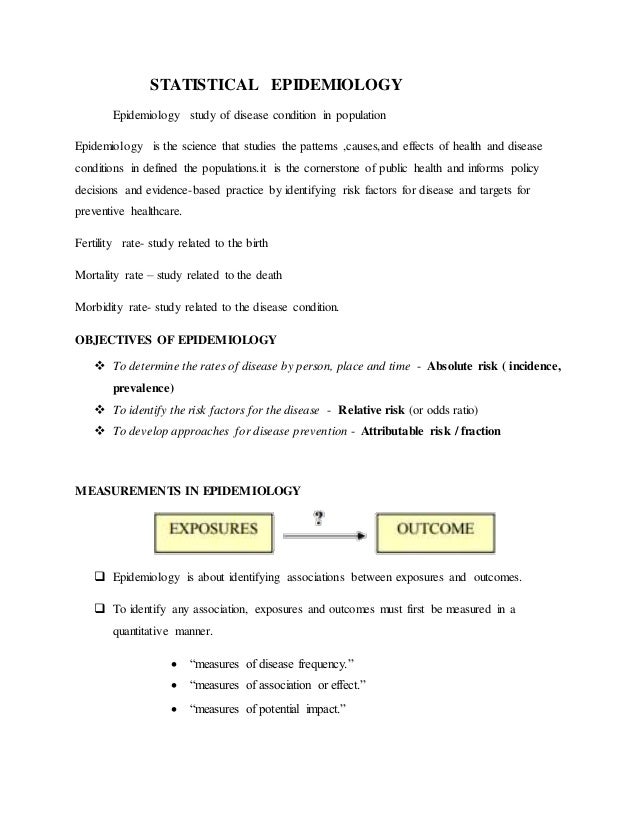
Reflecting the strong historical tie between epidemiology and medicine, formal training programs may be set in either schools of public health and medical schools. Some epidemiologists work 'in the field'; i. Epidemiologists can also work in for-profit organizations such as pharmaceutical and medical device companies in groups such as market research or clinical development.
From Wikipedia, the free encyclopedia. For other uses, see Epidemiology disambiguation.
For the journal, see Clinical Epidemiology journal. History of emerging infectious diseases. Health and fitness portal Medicine portal. A Dictionary of Epidemiology 6th ed. Retrieved 16 July The Challenge of Epidemiology: Issues and Selected Readings. Pan American Health Organization. A history of epidemiologic methods and concepts. Historic Developments in Epidemiology.
Jones and Bartlett Publishing, Background to Epidemiology" PDF. Retrieved 3 February Gro Harlem Brundtland, M. Geneva, Switzerland Talk, Washington, D. The battle against neonatal tetanus in the island of Vestmannaeyjar Iceland during the 19th century" PDF. Retrieved 19 April